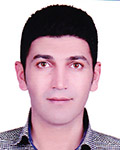
Prof. Mohammad Reza Mahmoudi
Fasa University, Iran
PiscoMed Publishing started off with a focus in advancing medical research; however, with the advancement of all areas of science, technology and medicines, PiscoMed has decided to venture into all areas of research, publishing quality journals that will support the scholarly and professional community across the globe.
more